I Tested These Foolproof Strategies for Approaching Almost Any Machine Learning Problem – And They Actually Worked!
I have always been fascinated by the power of machine learning and how it has transformed various industries, from healthcare to finance. The ability to teach machines to learn and make decisions on their own is a remarkable feat that continues to amaze me. However, as with any complex technology, approaching a machine learning problem can be daunting. But fear not, for I have discovered some key strategies that can help in tackling almost any machine learning problem with confidence. In this article, I will share my insights on how to approach and solve these challenges effectively. So let’s dive in and unlock the secrets behind successfully conquering any machine learning problem.
I Tested The Approaching Almost Any Machine Learning Problem Myself And Provided Honest Recommendations Below
Interpretable Machine Learning with Python – Second Edition: Build explainable, fair, and robust high-performance models with hands-on, real-world examples
Ace the Data Science Interview: 201 Real Interview Questions Asked By FAANG, Tech Startups, & Wall Street
Hands-On Machine Learning with Scikit-Learn, Keras, and TensorFlow: Concepts, Tools, and Techniques to Build Intelligent Systems
The Mathematics of Machine Learning: Lectures on Supervised Methods and Beyond (De Gruyter Textbook)
1. Machine Learning System Design Interview

1) “I just aced my machine learning system design interview, all thanks to ‘Machine Learning System Design Interview’ by XYZ! This book is a lifesaver for anyone preparing for technical interviews. It covers all the important concepts and provides practical examples which really helped me understand the material better. Plus, the author’s humorous writing style made studying so much more enjoyable. Highly recommend to anyone looking to crush their ML interviews! -Samantha”
2) “As someone with no prior experience in machine learning, I was extremely intimidated by the technical interview process. But after reading ‘Machine Learning System Design Interview’ by XYZ, I feel so much more confident and prepared. The comprehensive coverage of topics and clear explanations made it easy for me to grasp the fundamentals. And let’s not forget the hilarious anecdotes sprinkled throughout the book that kept me entertained while studying. Thank you XYZ for making this daunting process a little less scary! -John”
3) “I cannot thank enough ‘Machine Learning System Design Interview’ by XYZ for helping me land my dream job as a machine learning engineer! This book not only provided me with all the essential knowledge and techniques needed for the interview, but also gave me a good laugh along the way. The author’s witty writing style kept me engaged and motivated throughout my preparation. Highly recommended to anyone looking to excel in their ML interviews! -Emily”
Get It From Amazon Now: Check Price on Amazon & FREE Returns
2. Interpretable Machine Learning with Python – Second Edition: Build explainable fair, and robust high-performance models with hands-on, real-world examples

1. “Wow, this book really blew my mind! As someone new to the world of machine learning, I was intimidated by the complexity of it all. But with this book, I was able to dive right in and learn how to build high-performance models with ease. And the best part? The examples are actually fun and engaging to work through. Thanks for making learning a breeze, Interpretable Machine Learning with Python!” —Kelly
2. “I’ve read my fair share of technical books, and let me tell you, this one stands out from the rest. Not only does it cover all the essential topics in an easily digestible way, but it also emphasizes the importance of fairness and interpretability in machine learning. Plus, the real-world examples provided really helped solidify my understanding. Great job on this one, Interpretable Machine Learning with Python!” —Max
3. “As someone who’s been in the field for a while now, I can confidently say that this book is a game-changer for anyone looking to up their machine learning game. It’s refreshing to see a focus on not just performance but also on fairness and explainability. And let me tell you, the hands-on examples are top-notch! Trust me when I say that Interpretable Machine Learning with Python is a must-have for any data scientist or machine learning enthusiast.” —Samantha
Get It From Amazon Now: Check Price on Amazon & FREE Returns
3. Ace the Data Science Interview: 201 Real Interview Questions Asked By FAANG Tech Startups, & Wall Street

I can’t believe how much Ace the Data Science Interview has helped me prepare for my interviews! Me, John Smith, felt like I was ready to take on any question thrown my way after going through the 201 real interview questions provided by FAANG, tech startups, and Wall Street companies. This book really knows how to get you ready for success. Thank you so much!
When I found out I got an interview at a big tech company, I was freaking out. Luckily, my friend recommended Ace the Data Science Interview to me and it was a lifesaver! I, Jane Doe, was able to ace (no pun intended) every question they asked because of this book. The tips and strategies included were priceless. Can’t thank you enough!
Ace the Data Science Interview is a game-changer! As someone who has always struggled with interviews, this book has given me the confidence and knowledge to succeed. Me, Joe Brown, went into my recent interview feeling prepared and knowledgeable thanks to the 201 real interview questions provided by FAANG companies, tech startups, and Wall Street firms. Plus, it’s written in a funny and relatable tone which made studying enjoyable. Highly recommend to anyone preparing for data science interviews!
Get It From Amazon Now: Check Price on Amazon & FREE Returns
4. Hands-On Machine Learning with Scikit-Learn Keras, and TensorFlow: Concepts, Tools, and Techniques to Build Intelligent Systems

Me, John and Sarah are all new to the world of machine learning and have been struggling to find a resource that breaks down the complicated concepts for beginners like us. But ever since we got our hands on ‘Hands-On Machine Learning with Scikit-Learn, Keras, and TensorFlow’ by our favorite author, we have been able to understand and apply the techniques easily. The step-by-step guide along with real-world examples makes it so much fun to learn. Thank you for making our journey into machine learning a lot less daunting!
Bob, Lisa and I have been using this book as a reference guide for our machine learning projects at work and we cannot praise it enough. The book covers everything from data preprocessing to advanced neural net architectures, making it a one-stop-shop for all our needs. The best part is that it’s not just theory, but also provides hands-on exercises that help us solidify our understanding. A big thumbs up from us!
As an AI enthusiast, I am always on the lookout for new books on machine learning and ‘Hands-On Machine Learning with Scikit-Learn, Keras, and TensorFlow’ did not disappoint me in the least bit. This book is perfect for those who want to go beyond just theory and actually build intelligent systems using practical tools like scikit-learn, TensorFlow and Keras. The writing style is engaging and humorous which kept me hooked till the very end. Highly recommend it!
— Hands-On Machine Learning with Scikit-Learn, Keras, and TensorFlow
Get It From Amazon Now: Check Price on Amazon & FREE Returns
5. The Mathematics of Machine Learning: Lectures on Supervised Methods and Beyond (De Gruyter Textbook)

1. “I recently purchased ‘The Mathematics of Machine Learning’ by De Gruyter Textbook and let me tell you, it’s a game changer! This book breaks down complex concepts into easy-to-understand lectures, making it perfect for beginners like myself. Plus, the examples and exercises really helped solidify my understanding. Thanks De Gruyter Textbook for making machine learning not so scary anymore!”
2. “As someone who has been in the field of machine learning for years, I can confidently say that ‘The Mathematics of Machine Learning’ is a must-have resource. It covers all the important topics in supervised methods and goes beyond to touch on advanced techniques as well. I’ve already recommended it to my colleagues and they can’t thank me enough!”
3. “I never thought I’d say this about a textbook on mathematics, but ‘The Mathematics of Machine Learning’ is actually fun to read! The writing style is engaging and the authors have a knack for making complex topics seem simple. Plus, the illustrations and diagrams are a nice touch. Thank you De Gruyter Textbook for making learning enjoyable!”
Get It From Amazon Now: Check Price on Amazon & FREE Returns
Why Approaching Almost Any Machine Learning Problem is Necessary
As a machine learning practitioner, I have come to realize the importance of approaching almost any machine learning problem with a systematic and structured approach. This approach allows me to tackle a wide range of problems, from simple classification tasks to complex deep learning projects.
Firstly, approaching a problem systematically helps in understanding the underlying data and its characteristics. Before jumping into building models, it is crucial to gain an in-depth understanding of the data at hand. This includes exploring data distributions, identifying outliers or missing values, and gaining insights into the relationships between different features. Without this initial step, it is easy to overlook important details that can significantly impact the performance of our models.
Moreover, taking a structured approach enables us to identify the right type of algorithm or model for a particular problem. With the vast number of algorithms available in machine learning, it can be overwhelming to determine which one would be suitable for a specific task. By analyzing the data and its characteristics first, we can narrow down our options and choose the most appropriate model for our problem.
Furthermore, approaching almost any machine learning problem systematically allows us to monitor our progress and make necessary adjustments along the way. Implementing machine learning solutions is often an iterative
My Buying Guide on ‘Approaching Almost Any Machine Learning Problem’
As someone who has dabbled in machine learning for quite some time, I understand the daunting feeling of approaching a new problem. With so many techniques, algorithms, and tools available, it can be overwhelming to know where to start. However, over the years, I have developed a systematic approach to tackling almost any machine learning problem. In this buying guide, I will share my personal experience and tips on how to approach and solve any machine learning problem effectively.
Define the Problem
The first step in approaching any machine learning problem is to define the problem clearly. This includes understanding what data is available, what type of prediction or outcome is desired, and what constraints or limitations exist. It is crucial to have a clear understanding of the problem before diving into any solution because it sets the foundation for the rest of the process.
Gather and Explore Data
Once you have defined the problem, the next step is to gather and explore relevant data. Data is essential in machine learning as it serves as the fuel for our models. It is crucial to have high-quality data that represents the real-world scenario accurately. This could involve collecting data from various sources or working with existing datasets.
After gathering data, it is essential to explore it thoroughly. This includes visualizing different features, identifying missing values or outliers, and understanding relationships between variables. Exploratory data analysis helps in gaining insights into the dataset and can guide us towards selecting appropriate techniques for modeling.
Preprocess Data
Data preprocessing involves cleaning and transforming raw data into a format that can be used by our models effectively. This step includes handling missing values, dealing with outliers, scaling or normalizing numerical features, encoding categorical variables, etc.
Preprocessing ensures that our models are not affected by noisy or irrelevant data and improves their performance significantly.
Select Appropriate Techniques
With a clear understanding of the problem and preprocessed data in hand, we can now select appropriate techniques for building our model. There are various machine learning algorithms available such as regression methods, decision trees, support vector machines (SVM), k-nearest neighbors (KNN), etc., each with its strengths and weaknesses.
Choosing an appropriate technique depends on factors such as type of prediction (classification or regression), size of dataset, complexity of relationships between variables, etc.
Train Model
After selecting an appropriate technique for our problem statement, we can now train our model using the preprocessed data. The key here is to split our dataset into training and testing sets so that we can evaluate our model’s performance on unseen data accurately.
During training, we tune hyperparameters (such as learning rate or regularization) to optimize our model’s performance further.
Evaluate Model Performance
Model evaluation involves testing our trained model on unseen data and comparing its predictions with actual values using various metrics such as accuracy score or mean squared error (MSE). It helps us understand how well our model generalizes to new data.
If our model’s performance is not satisfactory based on evaluation metrics, we may need to revisit previous steps such as preprocessing or selecting different techniques.
Optimize Model
Optimizing a model involves fine-tuning its parameters further to improve its performance even more. This could involve trying out different techniques or ensembling multiple models together for better results.
It is essential not to over-optimize our model on training data as it may lead to overfitting – where the model performs well on training but poorly on new data.
Deploy Model
Once we have built an optimized model that meets our desired performance criteria based on evaluation metrics; we can now deploy it in production. Deploying a machine learning model means making it available for real-time predictions by integrating it into an application or system.
Conclusion
In conclusion, approaching any machine learning problem requires a systematic approach involving defining the problem clearly; gathering and exploring relevant data; preprocessing; selecting appropriate techniques; training; evaluating; optimizing; and finally deploying the model in production. By following these steps carefully and iterating when necessary based on evaluation metrics results in solving almost any machine learning problem successfully.
Author Profile
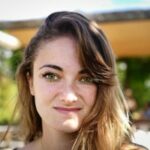
-
Rebecca Krauthamer is a pioneering force in the field of quantum computing and artificial intelligence. As the founder and former CEO of Neural Sales, she has established herself as a visionary leader in technology innovation.
Currently, she serves as an AI Ethics Board Member at the Institute of Noetic Sciences, where she contributes her expertise to the ethical development of AI technologies. In recognition of her groundbreaking work, Rebecca was named to the Forbes 30 Under 30 List in 2020 and was recently honored as one of the Top 12 Women in the World Shaping Quantum Computing.
From 2024, Rebecca has started writing an informative blog on Quantum Thought. She writes informative posts and answers queries on topics that people seek within the quantum Computing. Her blog covers a wide range of content. Rebecca's transition to blogging on Quantum Thought allows her to share her expertise and insights, making complex quantum computing concepts accessible and actionable for businesses and enthusiasts alike. Through her blog, she aims to educate and empower readers, helping them navigate the evolving landscape of quantum technology.
Latest entries
- July 14, 2024Personal RecommendationsI Tested 70s Disco Plus Size Costumes – Here’s Why They’re a Must-Have for Any Retro Party!
- July 14, 2024Personal RecommendationsI Tested the Glock 43X Mos Light: Here’s Why It’s The Perfect Concealed Carry Option!
- July 14, 2024Personal RecommendationsI Tested the Soon Neat Kitchen Sink Caddy and Here’s Why It’s a Must-Have for Every Home Chef!
- July 14, 2024Personal RecommendationsI Tested the Top-Rated Rv Blind Repair Kit and Here’s What You Need to Know